When I first started work on my doctoral degree, a project skirting the lines of neuroscience and molecular genetics, I had a mild idea of what I wanted from the science I was about to pursue and what I was getting into. And although I had a certain anticipation for it back then, I still managed to be surprised by how much of this doctoral work would require me to spends hours upon hours in front of my computer. As a traditionally trained biomedical scientist, it was an immense change from the laboratory benchwork I was trained for. There was a paradigm shift from my world of experimental protocols to a world of microscopes and image analysis. To a layman, this may not sound like a paradigm shift. A traditional life scientist does have a basic understanding of microscopes and of sample preparations. But what most life scientists probably do not foresee until they step into a laboratory to actually tackle cutting-edge research is that when you go from visualizing a fascinating biological phenomenon on a microscope, to capturing that image digitally with a camera, your science enters an interdisciplinary realm that leans much more in the direction of mathematics and computer science.
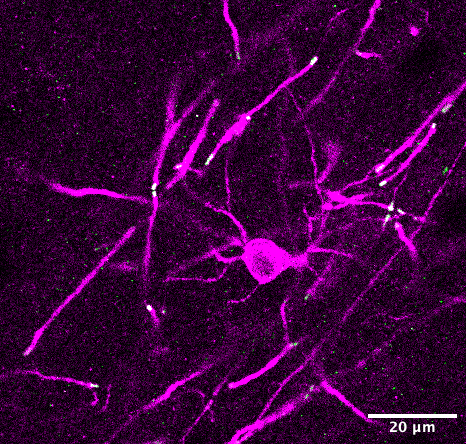
To put it simply, biological imaging transforms a biological sample from an observation under the microscope into pixels, pixel intensities, and pixel depth- all computational parameters. These parameters are exactly where the biological information of use is stored to be interpreted by life scientists. And yet here lies a bottleneck most life scientists do not truly comprehend they’re dealing with- understanding what these parameters really mean and how to handle them best for the optimal processing of their images is a prerequisite for correct analysis. When I started with my own image analysis work, I followed what I have now come to understand as the standard for most life science research groups around me- a senior group member shows you the basics of image processing which represent a standard protocol across the group. And these image processing standards can indeed prepare highly visually pleasing pictographs (that adorn the pages of many high impact journals). But, while this qualitative nature of image processing cannot be ignored, there is a more pressing need for processing to achieve correct preparation of these images for further analysis. However, most of the standard protocols for image processing in a research group do not truly account for experimental or even acquisition-based differences, even within replicates of the same sample, which make image processing a precarious task. For example, between two images which photograph a certain tissue sample, measurements of pixel intensities may look the same, and they may even be quantified as the same. Yet when one normalizes these numbers to the background intensities, the adjusted intensity measurements may switch completely.
Navigating these differences requires an acute understanding of the mathematical numbers that make up these images. To move beyond simple normalizations, and approach more complex mathematical functions on these images to derive complicated metrics (such as 3D reconstructions of images, morphometric quantifications, or fractal analysis of objects to name a few) life scientists have to dig deeper into the mathematics of analyzing bioimages and/or look towards a trained computer scientist who already understands the ins and outs of specialized image analysis software.
Perhaps the most adverse outcome of the improper understanding of the digital components of microscope images materializes in the incorrect analysis of images, which in turn brings about incorrect data and findings. Indeed, a quick search for academic paper retractions for image issues and manipulations brings up thousands of papers. These range from inconsistent data to image duplications to suspected errors. But barring obvious fraud, one does have to consider how much of a role erroneous image processing and analysis play in the production of such blunders. On the other side of the coin remains data- which is peer-reviewed and published- yet in some instances completely unreproducible. While, not limited to bioimage analysis, or even to the field of life sciences, the reproducibility crisis does remain a distasteful topic of discussion among academics. It is fair to assume that steps in correcting error-prone data analysis would probably lead to higher reproducibility of data.
Recently, I attended a microscopy and imaging course as part of my doctoral curriculum. In a room filled with approximately 60 life scientists, a question came up as to how many of the doctoral candidates in that room ever had the opportunity to attend an advanced image processing and analysis course as part of their pre-doctoral training. A meagre 3 hands shot up into the air. Among a room full of people who had to routinely undergo the task of taking images, processing them and analyzing them, five percent of them had access to formal training for it before they had to start working on projects that demanded it of them. Every other person in that room learned on the job. While picking up these skills as part of the doctoral training is not necessarily bad, it raises the question why, if the field of life sciences is increasingly incorporating methods from informatics with every passing year, does the training to prepare life scientists to face the heavy challenge of understanding this incorporation not start any sooner? Most avenues for such formal training on image processing and analysis also have an obvious drawback in their appeal to life scientists- they are largely organized by and for mathematicians and computer scientists, and their understanding of the subject matter is very different from the understanding of life scientists.
Microscopy and imaging indeed have a long history in the study of biology- from characterizing tissues, to bacteria, to cellular organelles. The earliest microscopes were designed by Anton van Leeuwenhoek in the mid to late 1600s with which he made the first known visual descriptions of bacteria and protozoans and pioneered the domains of microscopy and microbiology. Almost half a century later, microscopy and advances in imaging are still just as relevant today- the Nobel Prize in Chemistry in 2008 was awarded for the discovery of the Green Fluorescent Protein that is used to visualize samples under fluorescent light today, in 2014 it was awarded to scientists for their development of the STED microscope and in 2017 the award was dedicated to the development of the Cryo-Electron Microscope for studying frozen biological samples.
While the imaging of biological samples is first and foremost a qualitative examination of the sample, with the advent of digitalization the quantitative capabilities of image analysis have become more and more crucial. And yet, the utilization of these capabilities remains a challenge among life scientists. In the recent past there have been efforts to improve some of these challenges. The creation and maintenance of bioimage depository databases, such as the Electron Microscopy Public Image Archive (EMPIAR, 2013), the Image Data Resource (IDR, 2016), or the EMBL-EBI BioImage Archive (2019) with the aims of improving the reusability and reproducibility of biological images, are part of the attempts towards making the field of bio-imaging more approachable and understandable for life scientists. However, there still needs to be more work done to bridge the knowledge gap between life scientists and the increasingly prominent force of computational resources in the field of life sciences.
About the Author
Darshana Kalita is a 2nd year PhD student at Heidelberg University studying the brain, its heterogeneity and how glia fit into it. She is a science communicator on instagram under @science.lizaktzxy